Challenge Description
Multiple Sclerosis (MS) is a neurological disease that affects millions of people worldwide. In MS, different
area of the brain become inflamed, damaging the myelin, the fatty tissue that surrounds and insulate nerve
fibers.
Magnetic Resonance Imaging (MRI) is a fundamental tool to reach the diagnosis of MS and to monitor its
progression and response to treatments. Accurate segmentation of MS lesions is essential for volumetric
quantification of MS lesion load. However, manual selection of MS lesions on MRI scans is a very strenuous
and time-consuming task and sufficiently large data sets with accurate manual segmentation by experts are
still lacking.
Consequently, the development of methods capable of automatically segmenting MS lesions is an unmet
need and would represent a key step in advancing clinical management and optimizing treatment for people
with MS.
In conjunction with the ICPR 2024 conference, we propose a new lesion segmentation competition focused
on improving the accuracy of MS lesion segmentation in MRI.
We plan to provide participants with an extensively annotated dataset derived from a heterogeneous cohort
of MS patients, which contains both baseline and follow-up MRI scan of each patient, acquired at different
hospital.
MSLesSeg focuses on developing algorithms that can independently segment MS lesions of an
unexamined cohort of patients. This segmentation approach aims to overcome current benchmarks by
eliminating user interaction and ensuring robust lesion detection at different timepoints, encouraging
innovation and promoting methodological advances.
Main Contact
- Name : Alessia Rondinella
- Email : alessia.rondinella@phd.unict.it
- Address : Dipartimento di Matematica e Informatica Cittadella Universitaria - Viale A. Doria 6 – Italy.
Organizers
- Alessia Rondinella, alessia.rondinella@phd.unict.it (Department of Mathematics and Computer Science, University of Catania, Italy)
- Francesco Guarnera, francesco.guarnera@unict.it (Department of Mathematics and Computer Science, University of Catania, Italy)
- Sebastiano Battiato,sebastiano.battiato@unict.it (Department of Mathematics and Computer Science, University of Catania, Italy)
- Elena Crispino,elena.crispino@phd.unict.it (Department of Biomedical and Biotechnological Sciences, University of Catania, Italy)
- Giulia Russo,giulia.russo@unict.it (Department of Drug and Health Sciences, University of Catania, Italy)
- Francesco Pappalardo,francesco.pappalardo@unict.it (Department of Drug and Health Sciences, University of Catania, Italy)
- Clara Di Lorenzo,claradilorenzo@icloud.com (UOC Radiologia, Azienda Ospedaliera Garibaldi, Catania, Italy)
- Davide Maiomone,davide.maimone59@gmail.com (UOC Neurologia, Azienda Ospedaliera Cannizzaro, Catania, Italy)
Important dates
List of registered teams
Team Name | Organization/Institution | |
---|---|---|
1 | MSUniCaTeam | Dept of Mathematics and Computer Science, University of Cagliari |
2 | SJTU_SEIEE_2-426Lab | Department of Automation, Shanghai Jiao Tong University, Shanghai, China |
3 | LST | Laboratory of Imaging Technologies, Faculty of Electrical Engineering, University of Ljubljana |
4 | AthenaRC-DUTH | Athena RC in conjuction with the Democritus University of Thrace |
5 | UBHM | University of Bucharest, Helmholtz Munich |
6 | UGIVIA-UIB Team | University of the Balearic Islands |
7 | MadSeg | University of Wisconsin-Madison |
8 | BrainS | University of Brescia |
9 | NDSU REU ML | North Dakota State University, USA |
10 | Vishleshak | Working Professional |
11 | BeckLab | Icahn School of Medicine at Mount Sinai, New York, NY, US. |
12 | RVLab | Chungbuk National University/RVLab |
13 | M3S: MeetMIALMS | CIBM Center for Biomedical Imaging, Switzerland |
14 | Student | University of Cote d'Azur |
15 | ARTEAM | Altınay Robot Teknolojileri |
16 | PicusLabMed | University of Naples Federico II |
17 | ICAI | University of Malaga |
18 | MMS | TU Munich |
19 | BronTeam | John von Neumann Faculty of Informatics, Obuda University, Hungary |
20 | AIMH4Healtcare | ISTI-CNR, Pisa, Italy |
21 | INTELLIGO Labs | University of Verona |
22 | LA2I2F | University of Udine |
23 | Data Explorers | Data Scientist Professional working in the Data Field |
24 | Tequila | George Emil Palade University, Tirgu Mures, Romania |
25 | Golestan | Golestan university |
26 | UMD-PRG | UMD College Park |
27 | Genshin Impact Starts | Harbin Institute of Technology at Shenzhen |
28 | AdasLab - Applied Data Science Lab | UOC - Universitat Oberta de Catalunya |
An email with a proper link for training set download will be sent to each teams
Training-set structure
- Px (patient)
- Tx (timpoint)
- Px_Tx_T1.nii.gz (T1-weighted)
- Px_Tx_T2.nii.gz (T2-weighted)
- Px_Tx_FLAIR.nii.gz (FLAIR)
- Px_Tx_MASK.nii.gz (Ground-Truth segmentation mask)
- Tx (timpoint)
- Px_Tx_T1.nii.gz (T1-weighted)
- Px_Tx_T2.nii.gz (T2-weighted)
- Px_Tx_FLAIR.nii.gz (FLAIR)
- Px_Tx_MASK.nii.gz (Ground-Truth segmentation mask)
A link will be sent to the team email with a proper link for test set download
Test-set structure
- Px (patient)
- Px_T1.nii.gz (T1-weighted)
- Px_T2.nii.gz (T2-weighted)
- Px_FLAIR.nii.gz (FLAIR)
To ensure the Dice score is calculated correctly please use the following script DICE_EVAL.
All the instructions on how to use it are described inside the README file.
- Px_T1.nii.gz (T1-weighted)
- Px_T2.nii.gz (T2-weighted)
- Px_FLAIR.nii.gz (FLAIR)
All the instructions on how to use it are described inside the README file.
Upon completion of the challenge, participants are required to submit their results via email (from the registered email address) to the main contact (alessia.rondinella@phd.unict.it). The submission email should include a link to a repository with the following modality:
- Subject: MSLesSeg Challenge Results TEAMNAME
- The repository must contain a zip file to download named TEAMNAME.zip
- The zip file must be organized as follow:
- Directory DESC containing a PDF file with a brief summary of the segmentation approach used (1-2 pages)
- Directory MASKS containing a list of files named Px_PRED.nii.gz.
- 3D masks must have the same spatial resolutions (182,218,182) and orientations (X,Y,Z) of the Ground-Truth segmentation mask released during training phase
Only the last submission for team will be considered. Team with more than 3 submission will not be coonsidered
- Directory DESC containing a PDF file with a brief summary of the segmentation approach used (1-2 pages)
- Directory MASKS containing a list of files named Px_PRED.nii.gz.
- 3D masks must have the same spatial resolutions (182,218,182) and orientations (X,Y,Z) of the Ground-Truth segmentation mask released during training phase
Team Name | Organization/Institution | DICE SCORE | |
---|---|---|---|
1 | MadSeg | University of Wisconsin-Madison | 0.7146 |
2 | BrainS | University of Brescia | 0.7083 |
3 | M3S: MeetMIALMS | CIBM Center for Biomedical Imaging, Switzerland | 0.7079 |
4 | AdasLab | UOC - Universitat Oberta de Catalunya | 0.6974 |
5 | MMS | TU Munich | 0.6859 |
6 | LST | Faculty of Electrical Engineering, University of Ljubljana | 0.6783 |
7 | BeckLab | Icahn School of Medicine at Mount Sinai, New York, NY, US | 0.6754 |
8 | MSUniCaTeam | Dept of Mathematics and Computer Science, University of Cagliari | 0.6508 |
9 | Golestan | Golestan university | 0.6503 |
10 | LA2I2F | University of Udine | 0.6446 |
11 | UBHM | University of Bucharest, Helmholtz Munich | 0.6357 |
12 | UGIVIA-UIB Team | University of the Balearic Islands | 0.6101 |
13 | BronTeam | John von Neumann Faculty of Informatics, Hungary | 0.5683 |
14 | INTELLIGO Labs | University of Verona | 0.5471 |
15 | Student | University of Cote d'Azur | 0.4985 |
16 | ICAI | University of Malaga | 0.2351 |
Competition at ICPR 2024
General info
Our initiative aims to fill existing gaps in fully automated MS lesion segmentation by providing participants
with an extensively annotated MRI dataset derived from a heterogeneous cohort of MS patients.
Our dataset contains series of data of about 90 patients acquired at multiple timepoints. These timepoint
vary from 1 to 5 per patient. Thus, we have approximately 150 MRI data series (timepoints) in total. Each
timepoint consists of three different scan modalities: T1-w, T2-w and FLAIR.
This dataset was meticulously preprocessed and annotated on FLAIR sequences by our experts,
encompassing T1-w and T2-w sequences for comprehensive lesion characterization.
A notable strength of our initiative lies in the substantial number of patients and annotated scans, surpassing
publicly available datasets traditionally used for MS lesion segmentation. Furthermore, our dataset is
distinctively advantageous as it is representative of real-world scenarios, featuring authentic MS patients,
and is acquired "in everyday practice", reflecting a heterogeneous acquisition environment without
constraints.
The goal is to build an algorithm capable of generating an automatic segmentation of MS lesions for an input
MRI data series (T1-w, T2-w and FLAIR) not seen by the model under training.
It is planned to provide a competition website/platform that will be used for the duration of the competition,
which will remain active thereafter. The platform will contain a private area for participants; in this area
participants can download the training/validation/test set, upload 1-2 page summary describing the
methodology and results (segmentation masks obtained on the test set).
In this competition, only fully automatic methods allowed. All methods may provide an automatic
segmentation of the MS lesions.
The data used in the training phase is limited to that provided by the challenge in order to compare methods
in the same environment, so no additional data are allowed.
Since this is the first challenge, it is not possible to estimate the exact number of participants. Given the
recent interest in the context of multiple sclerosis lesion segmentation, we are confident that a significant
number of groups will participate in the challenge (about 10).
Furthermore, we plan to launch the first edition of the MSLesSeg competition at ICPR 2024, with subsequent
editions planned as an annual tradition.
We plan to ask each participant to produce a 1-2 page summary detailing their technical solutions to the
challenge. Participants who do not comply with this request by the deadline will be excluded from the final
rankings and awards.
Teams with the highest scores in the challenge ranking will be reviewed by the organizing committee and will
receive an electronic certificate of successful participation. The methods presented by these teams will also
be described in the challenge report paper.
The organizers of the challenge will provide the results of a baseline methods.
The organizing committee will publish a paper describing the competition process and results (including
rankings) of the challenge. The authors of the best selected works will be invited to submit their contribution
to a special issue of a valuable Journal.
Each participant may upload (during the upload time) a maximum of 3 result files; the best one will be
considered for the participant's ranking. Each participant must upload also a 1-2 page summary describing
the methodology before the deadline. After the deadline, the score will be calculated only for participant
which upload the 1-2 page summary and at least one result file. All results will be shown publicly on the
competition website, which will be updated during the open training round and announced on the final round
phases of the closed test round of the task.
Dataset
The dataset will be public. All the details about the dataset are available on the site used for the challenge.
The dataset contains MRI scans of patients acquired at different timepoints and labelled scans and has
received the approval of the ethics committee of the hospitals where the scans were acquired. The dataset
and the annotations will be under CC BY 4.0 license.
The competition cohort consists of patients diagnosed with multiple sclerosis who were clinically scanned
with MRI brain acquisition protocols at different hospital centers in the city of Catania, Italy.
All the data were acquired in the last 3 years, during brain MRI scans.
The data were acquired in different hospital centers with different 1.5 Tesla MRI scanners (about 3), with
different MRI protocols, then annotated by two expert raters and validated by an expert specialized in
neurology and multiple sclerosis.
The training, validation and test sets will be selected randomly from the dataset, to ensure that the
distributions of multiple sclerosis patients conform to the distributions of a real-world scenario.
All data provided during the challenge will be described on the challenge site.
Each case consists of T1-w, T2-w and FLAIR-w scans (extracted from whole brain MRI) of a patient, acquired
at several time-point, and annotations of the labelled MS lesions from the FLAIRs for each time-point.
All the annotation were produced manually by two know-context operators and validated by a multiple
sclerosis expert neurology. Both the annotators had professional experience on the task, while the
neurologist which validated the segmentation masks had many years of experience in multiple sclerosis MRI
evaluation.
MS lesion annotations of the dataset were performed using Jim 9, a commercial semi-automated
segmentation tool, the link to which can be found here: https://www.xinapse.com/j-im-9-software/
The process used to determine the annotations is as follows:
1. Each MRI was reviewed by an experienced neurologist specialising in multiple sclerosis. The neurologist
identified and located the MS lesions using his expertise in MRI interpretation.
2. Two know-context operators segmented each identified lesion in the FLAIR scan, using T1- and T2-
weighted scans for complete lesion characterisation. (MS lesions typically appear hyperintense in T2-
weighted and FLAIR scans and hypointense in T1-weighted scans). The labels were generated using the tool
mentioned above.
3. The neurologist verified the accuracy of the segmentations by visual inspection of the produced labels
together with the corresponding MRI scans, manually correcting any discrepancies if necessary.
Steps 2 and 3 were iterated until the neurologist was satisfied with the quality of the generated labels.
During the manual labelling process, we consider a possible source of segmentation error resulting mainly
from the existence of small MS lesions in MRI scans. Patients with multiple sclerosis often have numerous
small lesions, usually less than 3 mm, so that the precise identification of these small lesions becomes
difficult. To reduce potential inaccuracies, a validation process was implemented during the final data
verification phase, systematically on all segmentation masks. Lesions that could not be distinctly identified
on two consecutive slices were subsequently excluded from the segmentation masks.
Errors in the segmentation process can also depend on inter- and intra-operator variability. To address this
issue, the masks labeled by annotators underwent validation by the expert neurologist specializing in multiple
sclerosis.
We did an initial pre-processing on the MRIs: the original DICOM file was anonymised and converted to Nifti.
It was then registered with the standard MNI-152 template. Finally, skull-stripping was performed, in order
to extract only the brain tissue.
All the annotations were produced after the registration of MRI with MNI-152 template from the FLAIR
sequences, where the lesions appear hyperintense relative to the white matter. T1- and T2-weighted scans
were used to confirm the presence of the lesions.
Evaluation
Participants will develop their methods using the training/validation dataset provided in the first phase. At
the end of the first phase, they will evaluate the results of their method on the provided test set, which will
be released in the second phase.
Subsequently, participants will have to submit the results obtained on the test set (the predicted
segmentation masks) and produce a 1-2 page summary describing their proposed method.
The submission instruction will be available after the approval of the competition.
Participants' methods will be evaluated based on their accuracy in segmenting MS lesions, measuring how
well the predicted lesions from the proposed method overlap with the real lesions in the ground-truth mask,
using a metric based on overlap. This overlap-based metric will be used to rank the proposed methods. The
evaluation metric used will be the Mean Dice Score (DSC), which is a commonly used metric for evaluating
the performance of binary segmentation. It is particularly useful in medical imaging for tasks such as lesion
segmentation, where precise object delineation is critical.
The Dice score value is between 0 and 1, where 0 means that there is no agreement between the predicted
mask and the ground-truth mask, while a value of 1 means that the predicted mask and the ground-truth
mask are completely overlapping.
Specifically, we will calculate the Dice score for each segmentation mask generated by their proposed
method (one mask for each data series in the test set) using the following formula:
where ‘A’ denotes the predicted segmentation mask and ‘B’ the ground-truth mask. Here, the notation denote the cardinality of each set of voxel. The final performance ranking will be determined by the overall Mean Dice Score calculated across all data series in the test set.
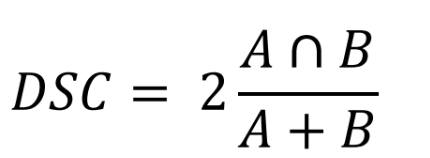
where ‘A’ denotes the predicted segmentation mask and ‘B’ the ground-truth mask. Here, the notation denote the cardinality of each set of voxel. The final performance ranking will be determined by the overall Mean Dice Score calculated across all data series in the test set.